AI for Improving the Reliability and Resilience of Industrial Fleets
To improve the fault diagnostics and prognostics accuracies in industrial automation, machine learning algorithms are being developed to extract fault and degradation signatures from multivariate system condition data of industrial fleets.
Factsheet
- Lead school School of Engineering and Computer Science
- Institute Institute for Data Applications and Security (IDAS)
- Research unit IDAS / Management Science, Innovation, Sustainability and Entrepreneurship (MSIE)
- Funding organisation SNSF
- Duration (planned) 01.03.2023 - 28.02.2026
- Project management Prof. Dr. Angela Meyer
- Head of project Prof. Dr. Angela Meyer
-
Project staff
Stefan Jonas
Dr. Albin Grataloup - Keywords machine learning, artificial intelligence, renewable energy, condition-based maintenance, fault detection, fault diagnostics, wind farms, industrial fleets
Situation
In industrial automation and condition-based maintenance, fleets of industrial assets are being sensor-monitored around the clock for the early detection and diagnosis of developing operation faults. Machine learning models have been proposed and are being utilized to automate these system health tasks in various types of industrial assets, such as wind turbines and photovoltaic power plants.
Course of action
A major goal in system health diagnostics and prognostics is that the machine learning models performing these tasks are accurate and generalizable to other systems in a fleet. This can be enabled by sufficiently large and representative training datasets. The data on which the model is being trained and the data that the model receives in operation should ideally originate from the same data-generating process and distribution, and be subject to the same tasks. Frequently, these assumptions do not hold. The objective of this project is to develop and assess machine learning approaches to overcome generalizability deficiencies that frequently arise and tend to deteriorate the performance of data-driven methods, for example, in fault detection and diagnostics.
Result
The approaches developed in this project aim to enable earlier commencement of required maintenance actions and more informed troubleshooting. In doing so, the results of this project will help improve the reliability and availability of industrial fleets and foster the resilience of the fleets’ maintenance strategies to changing operating conditions.
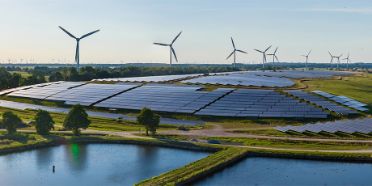